Bringing together more than 1,500 registrants, the 28th Annual Meeting of the American Glaucoma Society was the largest in the organization’s history. This year’s meeting was held in March 2018 in New York City.
For this article, I selected studies I believe have the potential to change glaucoma management today or in the near future, including a discussion on MIGS devices and a look at the intersection of artificial intelligence (AI) and medicine.
Microinvasive Glaucoma Surgery
The Hydrus Microstent (Ivantis; investigational use only; Figure) is a metallic intracanalicular scaffold that provides 90º of outflow effect. Thomas W. Samuelson, MD, presented 2-year data from the device’s FDA trial, in which 556 patients were randomly assigned to cataract surgery alone or with implantation of the Hydrus.1 Preoperative mean IOP was approximately 25 mm Hg in both groups. At 24 months, mean reduction in diurnal IOP was -7.5 mm Hg in the Hydrus group and -5.2 mm Hg in the control group. Seventy-seven percent of Hydrus patients achieved the primary study endpoint of a 20% or greater reduction in IOP compared with 58% of controls. At 2 years, 78% of Hydrus patients versus 48% of controls remained medication-free.
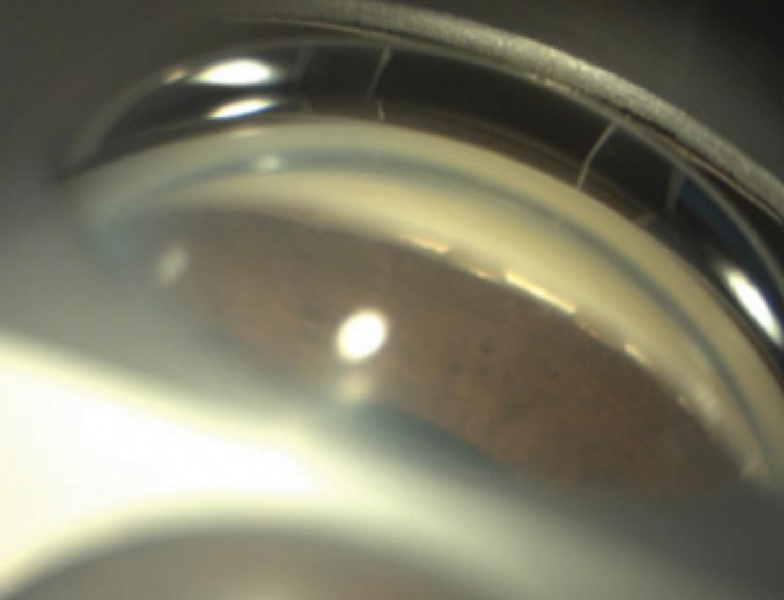
Figure. Hydrus Microstent in situ. Photo credit: Thomas Samuelson, MD.
Complications associated with the stent included transient hyphema in 1.1% of patients and obstruction of the device by peripheral anterior synechiae in 3.8% of patients. No cases of hypotony occurred. In the control group, 2.7% of patients experienced a significant IOP spike and 2.1% required glaucoma surgery.
Douglas J. Rhee, MD, presented the 12-month findings of a trial comparing the Hydrus Microstent with two current-generation iStent Trabecular Micro-Bypass Stents (model GTS100, Glaukos) as a standalone procedure.2 Investigators randomly assigned 152 patients without a history of incisional glaucoma surgery to receive the Hydrus or two iStents; approximately two-thirds of the patients were phakic. Mean unmedicated IOP was about 27 mm Hg on an average of 2.5 medications.
At 12 months, mean IOP was approximately 18 mm Hg in both groups. Patients in the Hydrus group required significantly fewer medications than those in the iStent group (mean, 1 vs 1.5). The Hydrus patients were also more likely than the iStent patients to be free of medication use (47% vs 24%). Few complications were observed. Device obstruction/adhesions occurred in 5% of the Hydrus group and 13% of the iStent group. One patient in each group required trabeculectomy.
ARTIFICIAL INTELLIGENCE
AI is the general concept of machines acting in a smart way. One application of AI is machine learning, which refers to a computer’s ability to learn without being explicitly programmed to do so. Deep learning systems are many-layered neural networks that can recognize patterns, a capability that is improved by using very large data sets. AI has received a great deal of attention in ophthalmology since the December publication of an article describing the use of a deep learning system to detect diabetic retinopathy in retinal images.3 [Editors’ note: EyewireTV covered the recent FDA approval of the first mobile AI platform to detect diabetic eye disease. You can view the episode here.]
Machine learning could also be used to recognize abnormal optic discs or to detect glaucomatous progression. At the AGS meeting, Osamah Saeedi, MD, reported on a project using 90,713 visual fields of 13,156 eyes from the Glaucoma Research Network collection.4 Investigators used available progression algorithms, including the Visual Field Index, to classify the fields as progressing or stable. The fields were used to train six different machine learning systems. Glaucoma specialists graded a subset of eyes for comparison.
The Visual Field Index had an accuracy (ability to differentiate progression from stability) of 95%. The machine learning classifiers had an accuracy ranging from 83% to 88%, a sensitivity of 76% to 85%, and a specificity of 89% to 94%. The investigators concluded that, in the future, machine learning could help clinicians determine which patients are experiencing disease progression.
- Samuelson TW. Results from the HORIZON trial: a multicenter, randomized study of a Schlemm’s canal microstent for reduction of IOP in primary open-angle glaucoma. Paper presented at: the 28th Annual AGS Meeting; March 1, 2018; New York, NY.
- Rhee D, Ahmed I, Fea A, et al. Prospective, multicenter, randomized comparison of stand-alone Hydrus versus two iStents for reduction of IOP in open-angle glaucoma. Paper presented at: the 28th Annual AGS Meeting; March 1, 2018; New York, NY.
- Ting DSW, Cheung CY, Lim G, et al. Development and validation of a deep learning system for diabetic retinopathy and related eye diseases using retinal images from multiethnic populations with diabetes. JAMA. 2017;318:2211-2223.
- Saeedi O, Boland M, D’Acunto L, et al. Development of a machine learning algorithm to determine visual field progression. Paper presented at: the 28th Annual AGS Meeting; March 2, 2018; New York, NY.