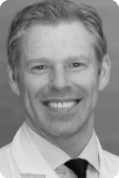
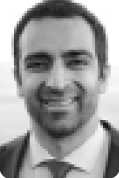
You may have seen or read about a paper published late last year in the Journal of the American Medical Association, describing the development and validation of a deep learning algorithm for detection of diabetic retinopathy (DR) from fundus photographs.1 In the study described in that paper, a Google artificial intelligence (AI) algorithm was able to interpret and grade fundus photographs with various stages of DR at least as accurately as a cohort of ophthalmologists, based on machine learning technology.
The algorithm’s success at diagnosing referable (moderate or above) DR was compared with the majority decisions of at least seven board-certified ophthalmologists grading more than 11,000 color fundus photos. In two image sets, the algorithm achieved sensitivity of 97.5% and 96.1% and specificity of 93.4% and 93.9%. Assuming an 8% prevalence of referable DR, these results yield a negative predictive value of 99.6% to 99.8%.
We were not authors on the Journal of the American Medical Association paper, but as physician consultants who worked with the Google team creating this technology, we want to address some of the common questions about this topic that we have heard from our eye care colleagues.
HOW DOES THE TECHNOLOGY WORK?
The Google deep learning algorithm used in this study of automated DR analysis was an advanced artificial neural network loosely modeled after the human brain. Artificial neural networks are computing system composed of many simple, highly interconnected processors. The many nodes or processors within the system each make simple calculations that are weighted and added together to produce the final output.
For this study, the Google system was initially trained by using about 120,000 color fundus photos, each labeled with a diagnosis by ophthalmologists. In the training phase, the system made a diagnostic “guess” on each image. It then compared its answer to the ophthalmologists’ labeled answer and adjusted the weights of each node, learning how to compute with the lowest possible diagnostic error. It did this again and again, hundreds of thousands of times. The system cannot simply memorize the diagnosis for each image, but rather is forced to learn broad rules that are more likely to generalize to future, unseen images. When this algorithm was validated in the study, 11,000 never-before-seen images were shown to the algorithm, and the results from the network’s analysis were compared with those from board-certified ophthalmologists.
HOW WILL THE TECHNOLOGY AFFECT PATIENTS?
In underserved populations with poor access to health care, there are great potential benefits to the use of machine-based automated diagnosis, including reduced costs and increased access to care. To this end, maintaining high specificity along with high sensitivity is critical. High sensitivity helps us to not miss patients with disease, but, in underresourced clinics, high specificity is also important to reduce overcrowding of clinics with patients without real disease. Google’s advanced algorithm is the first such system to perform well on both fronts.
In developed health care settings, there is also a place for automated diagnosis. Remember that large populations in the United States are not adequately screened for DR. This means that a new low-cost, highly efficient screening system could reach people who are currently not being screened. One might imagine screening kiosks in pharmacies and clinic lobbies. This type of service could lead to more patients gaining access to eye care. Also, if the quality of the system is sufficient, it might eventually serve as a diagnostic aid to eye care professionals, improving the efficiency of eye care delivery.
IS IT A THREAT TO PRACTITIONERS?
Eye care providers may react to the idea of AI in medicine with skepticism or fear. Some experts we have spoken with expressed clear worries that these types of technology may reduce the overall level of patient care, reduce eye care to “kiosk medicine,” or even become a threat to the livelihoods of providers who have invested so heavily in their medical education.
We believe that ophthalmologists and optometrists should view this technology with careful positivity. On one hand, it carries great potential. The Google technology has been developed to work synergistically with eye care providers. The potential benefits of an automated DR screening program include increased efficiency and coverage of screening (ie, algorithms are programmed to withstand repetitive image processing without fatigue), access to screening in areas without eye care coverage, earlier detection of referable diabetic eye disease, and likely reduction of overall health care costs through earlier detection and intervention—not to mention reduction of vision loss. As a result, eye care providers may gain access to more patients who need our unique skill sets, leaving the screening to more efficient technologies.
That being said, it is likely that this type of technology, in its final version, will cause changes in clinical focus. As the “guardians of vision,” ophthalmologists and optometrists must take leading roles in determining how best to integrate these advances to improve patient care. As with most new technologies, early adopters will likely play a role in the integration of AI. Herein lies the opportunity: to help shape this technology to be the very best for our patients.
WHAT’S NEXT?
Google has shown that its algorithm can diagnose and grade one disease in a study setting. We still need to see the algorithm’s real-world performance. Also, there are many other diseases to work on, as well as the critical aspect of catching the life-threatening issues sometimes seen in screening images, such as ocular melanoma. We are hopeful that clinical use of this type of technology is close. At this point, the goal for the technology is to improve access to low-cost, high-quality eye screening technology, with a focus on underserved populations to reduce the burden of vision loss in the developing world.
Previously, the question was, “Can a machine diagnose as well as a physician?” We are one step closer to knowing the answer to that question. We must ask now ourselves, as skilled specialists charged with preserving our patients’ vision, how do we use this technology to provide the best care for our patients?
- Gulshan V, Peng L, Coram M, et al. Development and validation of a deep learning algorithm for detection of diabetic retinopathy in retinal fundus photographs. JAMA. 2016;316(22):2402-2410.